Speech is the most natural method of communication among humans. The speech signals contain many traits that reflect suitable information from the speaker, including the identity, emotions, or the presence of diseases which alter the quality of life of patients. Automatic recognition of speech traits has many applications including the medical field; for instance, the detection, monitoring, and assessment of voice disorders allow the development of computer aided tools to support the diagnosis and evaluation/screening of patients; the recognition of emotions. Traditional machine learning methods to recognize speech traits are limited by their capability to process raw speech signals, i.e., without any preprocessing or any feature extraction. During several years, the deployment of suitable systems required the knowledge of an expert in a specific topic to obtain the most informative features to model the information from data, which consumes time and resources that are not always available. This project aims to develop and test different architectures of DNNs to perform the automatic detection of different traits in speech. Two different approaches will be addressed: (1) the raw speech signal will be considered as the input, and (2) several features will be extracted from the signals and those feature vectors will be the input of the system. We will compare both approaches in order to conclude to which extent it is possible to work only with the raw signal and obtain interpretable results such that can be used in the clinical practice. In this proposal, DNN-based methods will be considered to three different problems: (1) classification/detection of multiple voice disorders, (2) monitoring of patients, and (3) detection of emotions and other paralinguistic aspects from speech.
Deep Speech
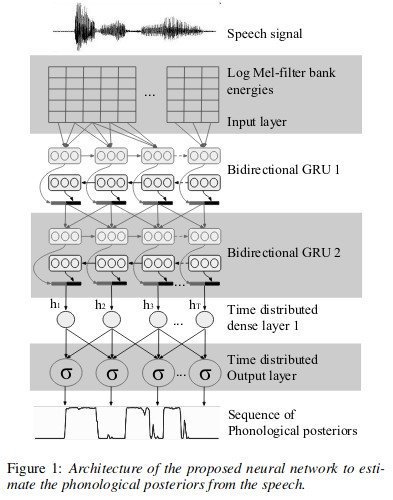